
"The training is guided by a target function that takes into account quantum effects," Liu told. In their study, Poulin and his colleague Ye-Hua Liu enhanced the performance of the 'initial neural network' by training it with data generated by numerical simulations. Past research has found that this 'initial neural network' does not work well in quantum settings, despite achieving better performance than random neural networks. In other words, it is possible to reinterpret the BP equations commonly used to decode LDPC codes as describing the initial setting of a neural network. The structure of these equations enables an exact mapping to a feed-forward neural network. This is due to a unique quantum feature referred to as 'error degeneracy," which essentially means that there are multiple ways to correct an error in quantum settings.Ĭlassical BP algorithms consist of of three simple equations. While BP decoders are commonly applied in a variety of settings, so far they have proved to be unsuitable for decoding quantum error-correcting codes. This was the perfect occasion to revisit my favorite open problem and use neural networks to decode quantum codes that had no previously known decoder." Recently, people have started to investigate the use of neural networks to decode quantum codes, but they all focused on a problem (decoding topological codes) that already had a number of good human-designed solutions. "This problem has been obsessing me ever since.
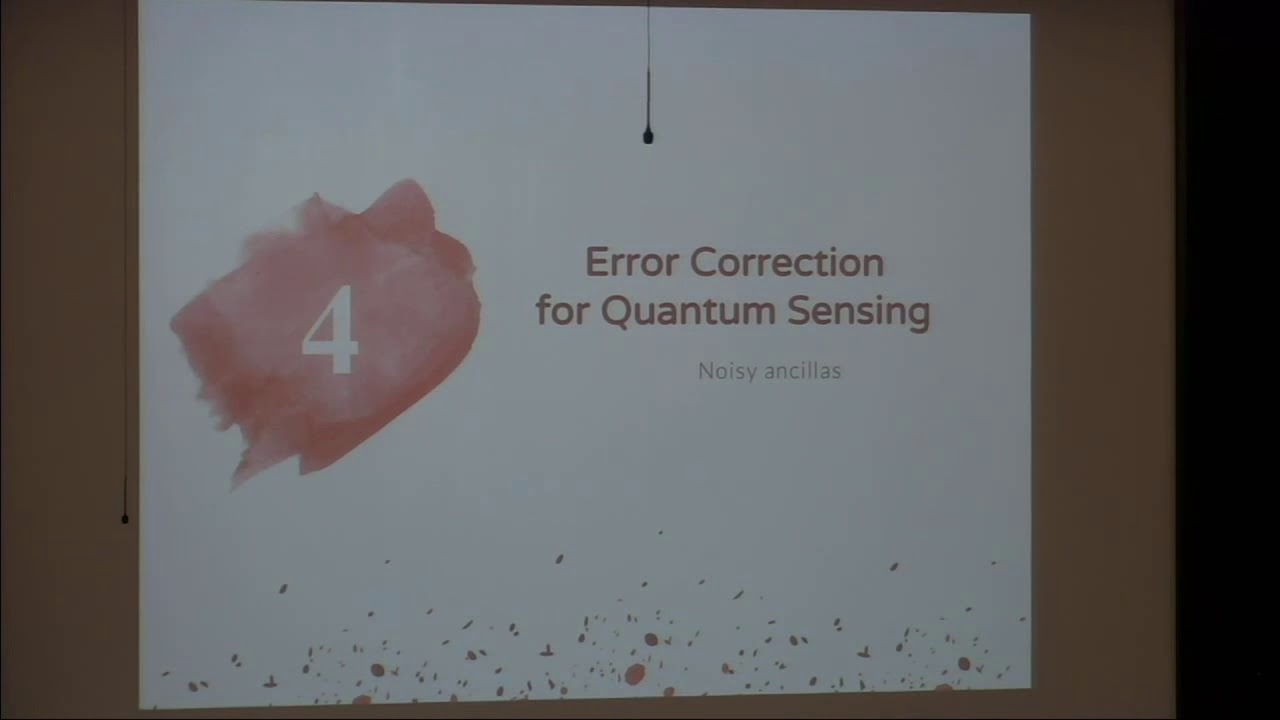
"Ten years ago, I wrote an article with Yeojin Chung explaining how standard decoding algorithms for LDPC codes, which are broadly used in classical communication, would fail in the quantum setting," David Poulin, one of the researchers who carried out the study, told.
